In the realm of artificial intelligence, the phenomenon of “grokking,” discovered somewhat serendipitously by OpenAI researchers Yuri Burda and Harri Edwards, presents a compelling narrative about the unpredictable and often perplexing nature of machine learning. Initially struggling to teach a large language model basic arithmetic, their accidental extension of the training period led to an unexpected breakthrough, challenging the traditional understanding of how AI learns.
This intriguing discovery led to a broader inquiry into why, in certain scenarios, AI models seem to suddenly grasp a concept after prolonged exposure—a behavior that deviates significantly from the expected learning patterns in deep learning. This phenomenon, termed “grokking,” has captivated the AI research community, raising critical questions about the learning limitations and potential of AI models.
As researchers delve into these anomalies, they confront the enigma of deep learning’s effectiveness. Despite its revolutionary applications, from Google DeepMind’s integration into consumer apps to OpenAI’s groundbreaking Sora model, the underlying mechanics of why these models work so well remain largely elusive. This gap in understanding poses not only a scientific challenge but also a pivotal concern for the future development and ethical deployment of AI technologies.
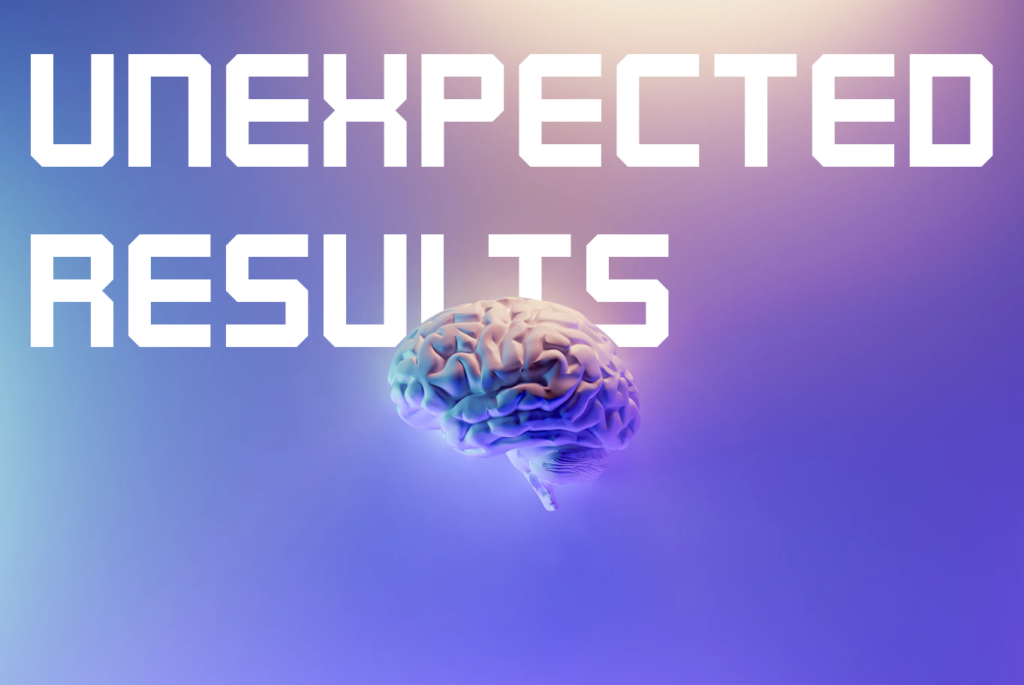
The perplexity extends to the core of machine learning—generalization. Large language models, such as GPT-4 and Gemini, demonstrate an astonishing capacity to generalize knowledge across languages and contexts, a feat that current statistical theories struggle to fully explain. This capability underscores the magic of AI, revealing a landscape where models can perform tasks they were never explicitly shown how to do, from solving math problems in multiple languages to adapting to entirely new scenarios.
Yet, as the field progresses, the limitations of current theoretical frameworks become increasingly apparent. The phenomenon of “double descent” exemplifies this, where models defy traditional statistical predictions by improving with increased complexity, suggesting a need to rethink the principles of generalization and model training.
Researchers like Mikhail Belkin from the University of California, San Diego, suggest that large language models might be tapping into underlying mathematical patterns in language, an idea that tantalizes the scientific community. However, the true nature of these models’ capabilities and the principles governing their learning processes remain subjects of speculation and ongoing research.
The challenge of understanding AI is likened to the exploratory nature of early 20th-century physics, a journey filled with experimental surprises and theoretical puzzles. As the field grapples with these mysteries, the quest for a comprehensive theory of deep learning becomes more urgent. Such understanding is crucial not only for advancing AI technology but also for addressing the ethical and safety concerns associated with its deployment.
In conclusion, as AI continues to evolve at a breakneck pace, the mysteries of grokking, generalization, and the inner workings of large language models remind us of the vast unknowns still to be explored. The journey to unravel these mysteries promises not just technological advancements but also a deeper comprehension of the principles underlying intelligence itself.
Sources:
- Discussion on the discovery and implications of grokking by Yuri Burda and Harri Edwards at OpenAI.
- Insights from AI researchers Hattie Zhou, Lauro Langosco, and others on the challenges and peculiar behaviors observed in AI models.
- Contributions from Mikhail Belkin and Boaz Barak on the theoretical gaps and potential explanations for AI’s capabilities.
- Examination of phenomena like “double descent” and the ongoing debates within the research community about the nature of AI learning and generalization.
Very interesting…!