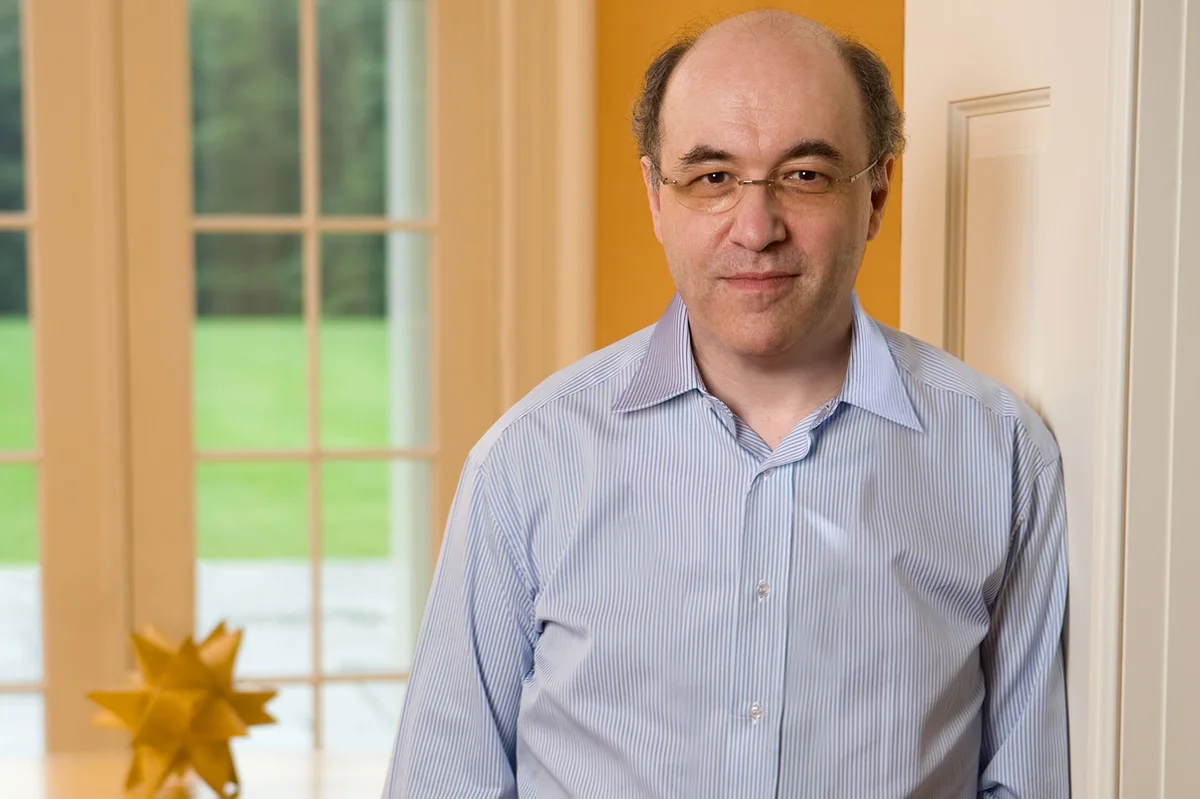
Link to the full article here.
From The Breaking AI, here is a succinct overview of Stephen Wolfram’s insightful article on the interplay between AI and science: While AI has demonstrated significant potential in various aspects of scientific research, it is crucial to recognize its inherent limitations, especially in the face of computational irreducibility. In this TL;DR, we distill the essence of Wolfram’s exploration into AI’s capabilities and constraints within the scientific domain, emphasizing the synergy between AI’s pattern recognition strengths and the indispensable role of human ingenuity in navigating the complex landscape of scientific discovery.
While there’s enthusiasm about AI’s capacity to “solve” science, given its achievements in generating human-like solutions and predictions, the reality is nuanced. AI’s strength lies in its ability to leverage computational reducibility, capturing regularities in a manner aligned with human cognitive processes, and offering new avenues for exploration and prediction within these bounds. However, AI’s effectiveness is inherently limited by computational irreducibility—where the deep, unpredictable complexity of systems resists simplification.
AI excels in scenarios where approximate solutions are acceptable, aiding in identifying patterns, suggesting research directions, or managing large data sets where traditional scientific methods may falter due to the depth of computation needed. Yet, when precision and formalization are paramount, AI’s approximations and “black-box” nature fall short, underscoring the irreplaceable value of human-led scientific inquiry and the use of computational language for deeper, more precise explorations.
The future of AI in science looks promising when considered as a complementary tool that augments the human capacity for discovery rather than as a standalone solution. By marrying AI’s pattern recognition capabilities with the precision and formal rigor of computational science, researchers can navigate the complexities of computational irreducibility more effectively, opening new frontiers for exploration and understanding in science.